Drilling - A Data Analytics Approach to Energy & Safety Improvement
About | Team | Roadmap | Challenges | Highlights | Contact |
About
The Drilling Data Analytics Approach to Energy and Safety Improvement project is a collaboration with the oil and gas industry to develop solutions to specific problems or “challenges” in the drilling industry.
The project uses a data-driven approach, agile methodology, with consistent industry feedback to make systems more efficient. Improved efficiency means reduced drilling time (cost savings), less energy, and fewer carbon emissions.
The project’s multidisciplinary team includes members from industry, consultants and academia, including research engineers, postdoctoral fellows, co-operative undergraduate and graduate student interns with experience in computer science, petroleum and computer engineering, and geoscience.
Drilling operations generate large amounts of data which can be analyzed in order to provide insight for:
- Real-time drilling optimization
- Automated and more efficient drilling workflows
- Enhanced data-driven decision making
- Early identification of drilling mishaps
- Cost savings from reduced drilling time
- Improved drilling safety
The project’s primary objectives include:
- Analyse drilling data using conventional, statistical, and artificial intelligence (AI) methods to understand how to make drilling operations more energy efficient and safe.
- Predict how and when drilling operations become inefficient or operate outside of acceptable standards.
- Educate students in the digitalization of offshore oil and gas operations.
- Recommend future technical and strategic project direction for energy and safety improvements, and educational requirements in drilling data analytics.
Meet The Team
The Multidisciplinary team is led by:
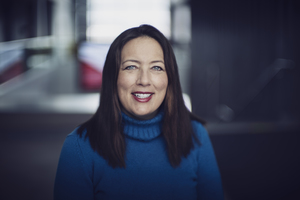
Principal Investigator | Professor, Process Engineering
“Currently, determining the type of rock is a manual process. Our team is using machine learning and computer vision to analyze data in real time to make predictions. Time is money and safety. If you can predict what is coming, you can drill at the right speed."
B.Sc. (Eng.) (University of New Brunswick), M.Eng., PhD (Waterloo), P.Eng.
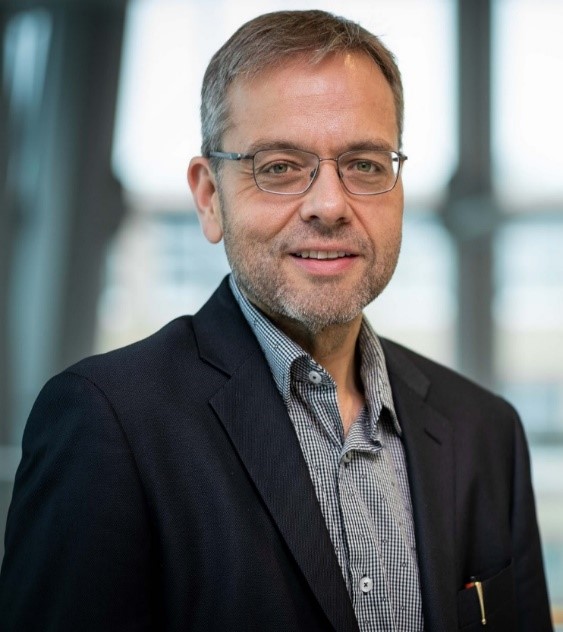
Co-Principal Investigator | Professor, Dept. of Mathematics & Statistics
“The appeal of the work is a delicate balance between relevance - something the industry partners are interested in - and academic rigour. Walking that tightrope between publishing the work and satisfying industry partners is appealing and I’d love to see more faculty members doing this kind of work."
PhD (Simon Fraser), M.Sc. (Simon Fraser), B.Sc. (Hons) (Memorial)
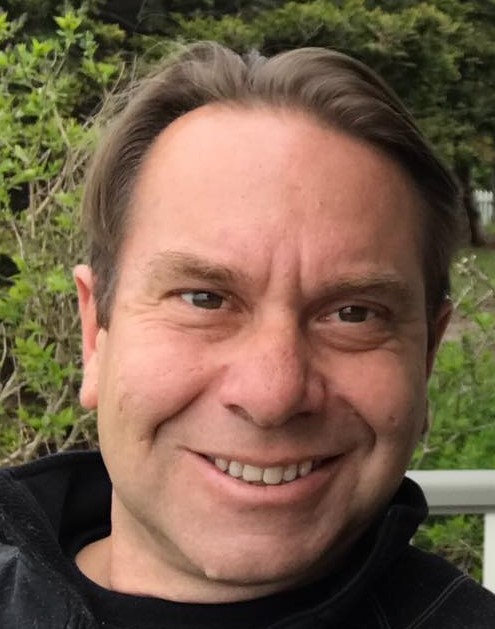
Technology Consultant | ACBBTech
"The drilling industry has been built on the provision, and interpretation of data to optimize, monitor and safely execute the drilling process. With the implementation of machine learning (ML) and artificial intelligence (AI), we can now implement industry best practices through expert-verified analytics and translate them into ML or AI. This approach helps offset knowledge gaps as many experts retire, and new employees face a steep learning curve, also known as "The Great Crew Change". Future automation and viability of the industry would not be possible without such efforts. Memorial has brought together a team that is diverse in both discipline and culture to succeed in bringing new solutions using AI and ML to many drilling challenges."
BSc. (Memorial)
Partners
Thank you to our partners and collaborators for their guidance and support of the project especially the Newfoundland and Labrador Department of Industry, Energy and Technology who supported the research through the NL Offshore Oil and Gas Industry Recovery Assistance Fund.
Roadmap
The project’s roadmap used an agile approach consisting of four phases.
The initial phase involved working with academic and field expects to prioritize specific drilling problems/challenges in the oil and gas industry. The project’s focus then shifted to solving these challenges through extensive drilling data analysis in order to develop quality solutions. Proposed solutions were shared with industry partners in order to obtain operators’ feedback. This critical point of the agile approach generated comments that added value to the final phase - improve based on feedback. Incorporating these improved solutions will lead to improved safety and energy efficiency during drilling activities, and further highlighted the importance of digitalization in the oil and gas industry.
Challenges
Identified below are some of the problems/challenges identified in the drilling industry and the developed methods from the project’s research.
Automated Drill Bit Cutter Damage Detection Using 2-D Images
The drilling industry has been longing for a consistent and accurate approach for evaluating drill bit wear and damage. Traditionally a manual and subjective approach recognized by the Society of Petroleum Engineers, and the International Association of Drilling Contractors, a challenge to academia was issued for a solution. The team at Memorial used computer vision and machine learning to recognize and evaluate the cutting structure from thousands of photographs. A web-based application was developed for the users to automatically capture and characterize the cutting structure, so drillers can better advise running procedures in response to the damage profile, as well as improve the bit selection process. The application stores the evaluation and retains its process for enhanced evaluation periodically. This results in improved rate of penetration, and reduced handling time as well as costs associated with the number of bits utilized for drilling activity.
Image of damaged drill bits provided by the Society of Petroleum Engineers
This video gives a brief overview of the functionality of the proposed method.
Drilling Parameter Search Space Prediction Using Machine Learning
Drilling efficiencies are influenced by the choice of values for weight on bit (WOB) and rotary speed (RPM). Hence, using appropriate values leads to improved drilling efficiency. Automatic drillers typically use set points of WOB and RPM values that may not be optimal in terms of minimizing mechanical specific energy. The research developed machine learning methodology using lithology, inclination, and dog leg severity that predicts WOB and RPM values to optimize mechanical specific energy. A more efficient drilling process means reduced drilling times (cost savings), less energy and lower carbon emissions.
Example result. Green box indicates recommended RPM/WOB region
Drilling Roadmap Optimization
To drill efficiently, a driller selects the proper control actions by reacting to observations and by following a prepared roadmap. Currently, road mapping is a manual and labor-intensive process. Response to problems while drilling is heavily reliant on the experience of the driller and the team that prepared the road map. Automatic driller systems are unable to react proactively to drilling dysfunctions, which can cause idle time. Therefore, a modular system was developed consisting of: i) supervised machine learning that infers the rock strength from surface drilling data, ii) a fuzzy logic system that relates the determined rock strength to a depth of cut limit that would prevent the bit cutters from being overloaded, and iii) a deep learning forecasting model that predicts changes in rock strength ahead of the bit based on offset well data. The modules are intended to provide real time automated/automatic driller set point recommendations and can also be used with offline data during the planning phase to make the road mapping process easier. This improved drilling efficiency method translates into reduced operating costs and lower carbon emissions.
Roadmap Optimization Concept
Vibration while drilling is a leading cause of downhole equipment failure. 2-10% of well costs are due to equipment damage caused by vibrations. There are three vibration modes that occur while drilling; axial vibration along the axis of the bit, torsional vibration, around the center of rotation of the bit, and lateral vibrations, which subject the bit and downhole equipment to bending stress. The three modes are coupled with bit rock interactions. A model to estimate drilling forces as the bit starts to rotate, moving until it contacts the rock and starts drilling was developed to evaluate the level of vibration generated by the recommended depth of cut generated by the roadmap optimization system. Future work will include performance indicators from the model in the fuzzy logic system to generate better depth of cut estimates.
Machine Learning Based Rock Strength Prediction using Offset Data
Drillers currently rely on a manual reactive approach to determine rock characteristics before the drill bit enters a new formation. The time lag between manual interpretation and response, frequently affects drilling performance in terms of drill bit failure, poor rate of penetration and drilling dysfunctions. By using machine learning methods, rock strength predictions can be made prior to drilling, depth shifting can be automated and formation tops can be determined based on offset wells and drilling behaviour. Machine learning can also be used to improve geosteering, and well completion. The estimation of rock strength ahead of time using data from offset wells can provide better control on drilling parameters. This would improve drilling performance and reduce downtime, which ultimately reduces energy consumption and associated carbon emissions.
Effective rock strength of a well in the Jeanne D’Arc basin located offshore Newfoundland & Labrador
The following animation shows a sonic log generated unconfined compressive strength (UCS) and corresponding drilling strength (Es). In normal drilling conditions, UCS and Es follow almost similar trends with offset in magnitude. However, in certain cases, Es shows an abnormal peak where UCS remains stable. These peaks can be an indication of drilling dysfunctions. Any abnormal peaks in Es are the zone of interest in drilling and need to be investigated in detail regarding rock strength behaviour and drilling dynamics.
Data-Driven Prediction of Drilling Strength Ahead of The Bit Based on Real-Time Drilling Data
In this challenge, a method is proposed based on Long Short-Term Memory (LSTM) neural networks. LSTM models are trained using drilling data from the offset wells. For each offset well an LSTM model is trained. In the prediction phase, the real-time data from the target well are fed into all LSTM models and the average of their output is used as the ultimate predicted value. The performance of the method is enhanced by giving a larger weight to the samples near the formation transition zones, hyperparameter optimization by PSO, and a post-processing technique.
The following animations show the predicted and actual drilling strength profile in the target well. The progress of the lines shows the concept of prediction ahead of the bit. In other words, the prediction line moves 3 feet ahead of the actual line. As shown, the actual line follows the same trend as that of the prediction line, indicating that the proposed method is accurately predicting the drilling strength ahead of the bit. The first animation is for the prediction before post processing is applied and the improvement due to the application of the post-processing technique is realized in the second animation.
Potential Environmental Impact of Implementation
The increase in rate of penetration (ROP) predicted by the developed methods would lead to decreased drilling time. Shorter drilling time implies less energy used. To get an idea of how these data-driven solutions might reduce GHG emissions, it was assumed that the application of the methods would amount to a 10% gain for ROP or reduction of 10% non-productive time, and would be applicable to the 12 ¼” and 8 ½” sections of hole only. Applying this to 24 studied wells from the Hebron field, offshore NL, this ideally would lead to an emissions reduction of approximately 25,000 tonnes CO2 eq.
Highlights
Congratulations to the team, Dr Lesley James, Alan Clarke, Hanoor Singh, Donald Hender, Abdulbaset Ali and Daniel Kelly, who placed second at the SPE-DUPTS competition. This international competition challenged teams to develop an algorithm to identify the root cause of drill bit failure from bit images. The challenge entailed using artificial intelligence, machine learning, and data science solutions to diagnose the fundamental cause of bit failure automatically.
Milestones
Contact
Faculty of Engineering and Applied Science
Memorial University of Newfoundland
St. John's, NL
https://www.linkedin.com/company/hibernia-enhanced-oil-recovery-group/